
حقيبة يد نسائية فاخرة ذات سعة كبيرة ، حقيبة عمل ، حقيبة دانتيل ، حقيبة كتف ، حقيبة ماسية ناعمة|حقائب الكتف| - AliExpress

سعر حقيبة جلدية رسول للرجال والنساء ، حقيبة كتف للرجال ، هدايا عمل جديدة للبنين في سن المراهقة ، حقيبة عمل المكتب للمنافسين التنفيذيين ، نصف رفرف فوق خمر براون حقيبة حقيبة
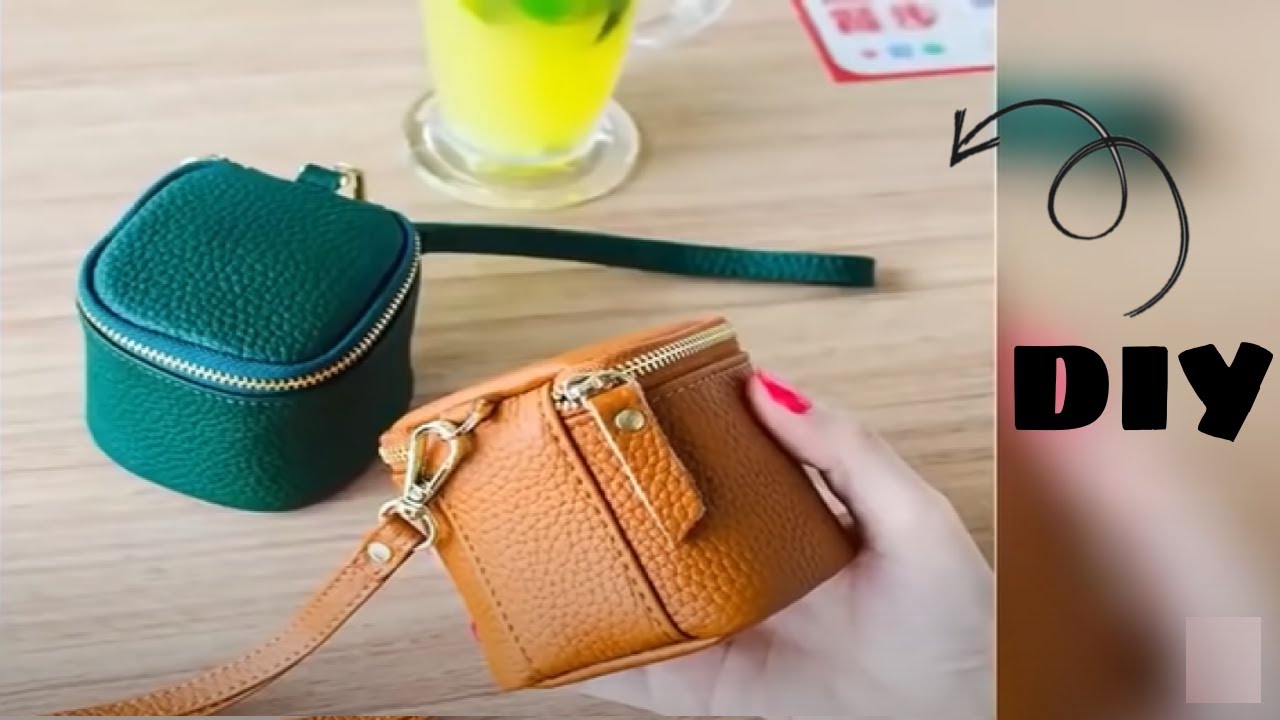
طريقة عمل شنتة نسائية / طريقة عمل حقيبة يد صغيرة / مقلمة جلد / حافظة مكياج /طريقة عمل حقيبة مكياج - YouTube
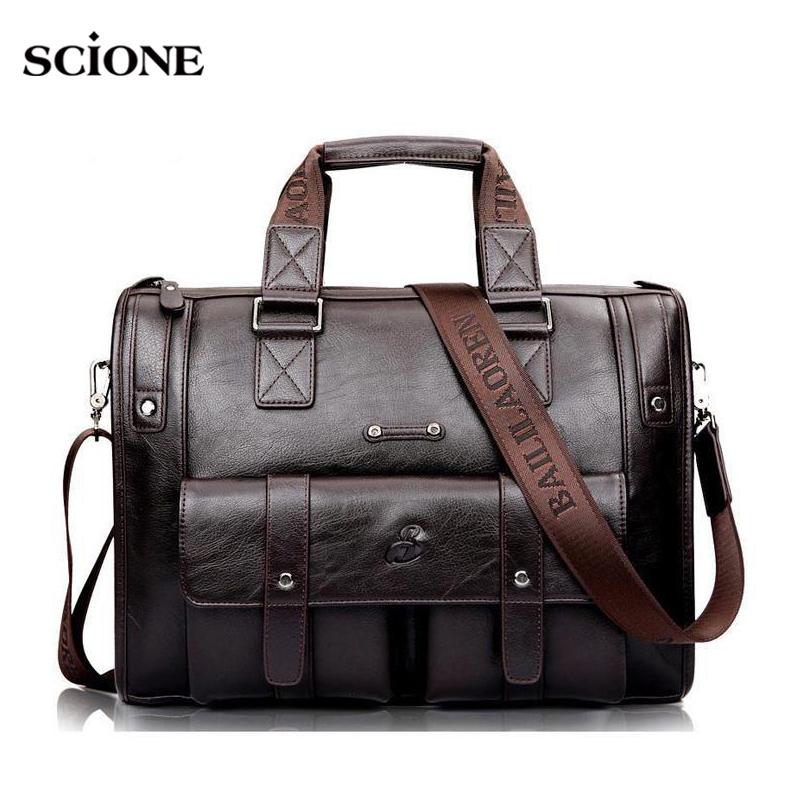
رجل جلد أسود حقيبة عمل حقيبة يد رسول ذكر قديم كتف كبير كمبيوتر محمول حقيبة سفر: الشراء بأسعار منخفضة في متجر Joom الإلكتروني

نساء بنات مباركة قماش حمل حقيبة يد حقيبة عمل حقيبة تسوق حقيبة شاطئية هدية للأصدقاء دروبشيبينغ|حقائب التسوق| - AliExpress

حقيبة كمبيوتر للنساء 15.6 عمل كمبيوتر حقيبة يد مكتب, بيج, 15.6 inch: اشتري اون لاين بأفضل الاسعار في السعودية - سوق.كوم الان اصبحت امازون السعودية
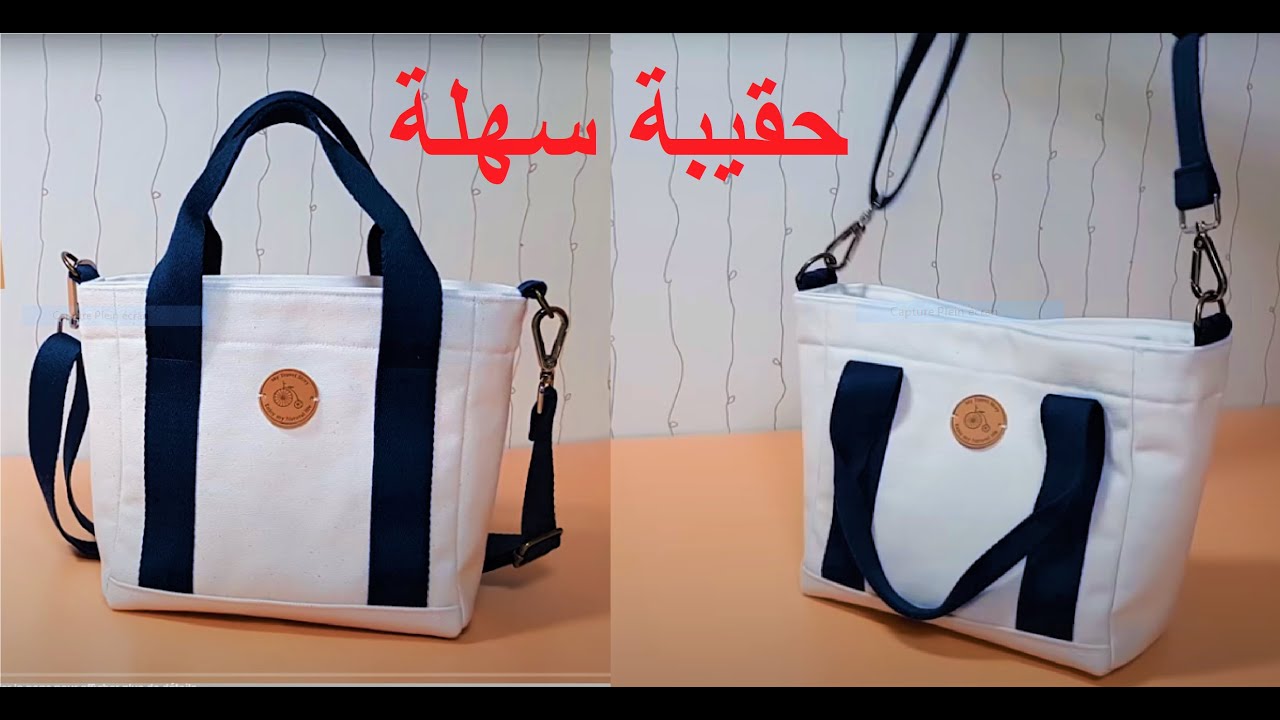
طريقة صنع حقيبة يد بالقماش - إصنعي حقيبتك بلونك المفضل - مشروع مربح 😍 How to Make a Bag at home - YouTube

حقيبة لابتوب للنساء 15.6 كمبيوتر عمل حقيبة حمل حقيبة مكتب, بني, 15.6 inch,: اشتري اون لاين بأفضل الاسعار في السعودية - سوق.كوم الان اصبحت امازون السعودية

حقيبة الأمتعة مرسومة باليد أصفر حقيبة يد التوضيح حقائب اليد في رحلة عمل, التوضيح, حقيبة التخزين, ناحية PNG وملف PSD للتحميل مجانا
